The Future of Personalized Medicine: Predictive Analytics
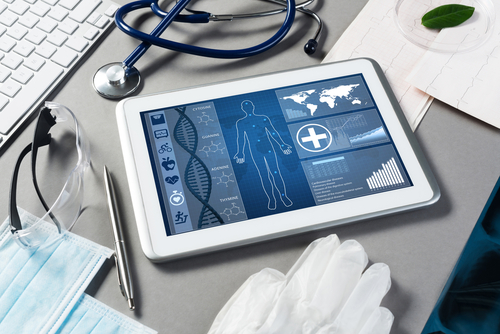
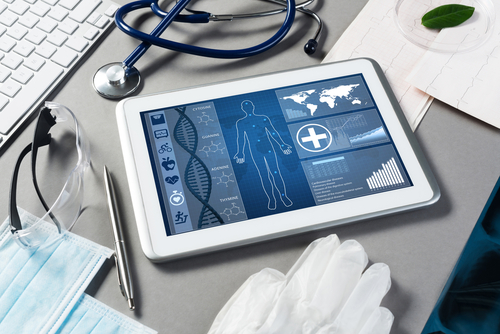
What are the chances a cancer patient lives after brain surgery?
How likely will a Covid-19 patient be readmitted to intensive care?
What is the likelihood that an elective procedure results in the loss of a limb?
Much of medicine has always been about anticipating and reducing patient risk, but physicians today have more tools than ever to answer medicine’s tough questions.
Thanks to statistical techniques like data mining and machine learning, we now have the ability to analyze current and historical data to forecast the likelihood of future events.
Welcome to the era of predictive analytics
In predictive analytics, algorithms are used to forecast a range of likely scenarios across an array of fields such as finance, heavy industry, retail, among many others.
In financial services, machine learning identifies and prevents fraudulent claims. In retail, employees utilize similar tools to understand what motivates their customers. And in the public sector, transit officials compile large swaths of commuter data to anticipate where riders are most likely to disembark.
Health care is no exception. In fact, it is fertile ground for predictive analytics
Of all the arenas in which predictive analytics is implemented, health care is expected to see the highest annual growth rate of all the others. According to current models, predictive analytics in health care could surge 36% between 2018-2025.
The explosion of health data in recent years — spurred by the rise of wearables, fitness apps and digital systems — is not only a boon for the digital health market, it plays a vital role in modern medicine, helping physicians make better informed medical decisions.
Physicians have always been tasked with making educated choices based on their experience and education — but they have never been able to do so with complete certainty. Doctors, after all, are human. Predictive analytics, on the other hand, enable physicians to make decisions backed by something more concrete, like mathematics.
The quantitative nature of predictive analytics offers a shield against human bias and can provide insights where the human mind may experience limits
But how does it actually work?
Predictive models make assumptions based on what has happened before and what is happening now. If incoming data demonstrates a shift, the impact on the likely future outcome will be recalculated. It’s this constant inflow of fresh data which ensures the high accuracy of predictive analytics.
Another advantage of predictive analytics is the prominent role it plays in personalized medicine. By examining a patient’s medical profile, we can create a highly-personalized drug program which is supported by big data.
At Datawizard, for instance, we consider a patient’s unique characteristics such as their biochemistry, lifestyle, lab analysis, clinical history, drug to drug interactions and genome. One of our key products in this area, Drug-PIN, is aimed at analyzing the effectiveness of a drug for each patient, highlighting the likely risks of adverse side effects for drug combinations too. Furthermore, Drug-PIN offers patients alternatives to high-risk drugs and may suggest the elimination of certain drugs altogether, allowing for fine-tuning of the patient’s therapy and reducing the overall risk of undesirable interactions.
While big data provides more accurate modelling on the effectiveness and combination of drugs, it also can provide a window into individual mortality rates. A patient’s risk of death in a medical operation, for instance, can now be determined by his current condition, medical history, and drug prescriptions. Big data can also offer a window into which patients are likely to face a number of other health conditions. The University of Pennsylvania, for instance, uses predictive modelling to identify patients who are most likely to experience septic shock 12 hours before the condition starts.
While it is virtually impossible for one health practitioner to manually analyze all the information out there, predictive analytics can demonstrate hidden patterns and unknown correlations, helping physicians find treatments for even the rarest diseases and unique cases. Predictive analytics can therefore provide a wider picture but also a more focused view.
The practice is becoming so widespread in health care, that even health insurance companies are turning to predictive analytics in order to measure risk.
Still, for all the benefits that predictive analytics provide, there are drawbacks
For one, technology’s fast pace can be hazardous. While machine learning tools offer speed, they don’t embody the inquisitive nature of the human mind.
Humans can postulate moral hazards; algorithms cannot. Caregivers have the propensity for compassion; algorithms do not. And they don’t have the propensity to consider the bigger picture or a patient’s unique set of circumstances either.
What algorithms do provide, however, are mathematical predictions backed by large quantities of data. Combined with the power of the human mind, new medical insights for the wider population as well as the individual can be realized.